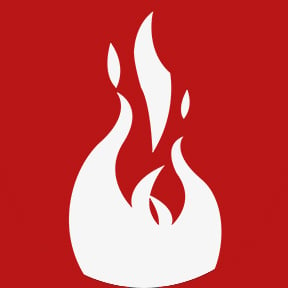
How Artificial Intelligence (AI) Can Be Used in Asset Management
Enterprise Asset Management (EAM) has been in a state of digital transformation for many years now. However, with the rise of new technologies, like artificial intelligence (AI), the push to digital has become even stronger.
For organizations, implementing AI into daily processes is a companywide initiative.
While AI is on the rise, what AI is and how to implement it into asset management can still be a large mystery for many.
In this article, we will walk through an explanation of AI in asset management, ways it can be used to enhance your processes, and benefits of implementing AI in your EAM.
Understanding Artificial Intelligence (AI) in Asset Management
Artificial Intelligence (AI) is a computer-generated simulation of human intelligence. It is designed to learn, reason, problem solve, perceive, understand languages, and interact with environments like the human brain.
AI learns from data using algorithms that detect patterns. It builds flexible models tailored to tasks by ingesting examples. These models allow AI to exhibit intelligence by generalizing its experience to apply learned patterns to new scenarios, continually improving with more data.
Most industrial companies have a wealth of operating and maintenance data in historians and EAM systems along with a long list of operating and maintenance challenges – creating a perfect scenario for AI applications.
6 Ways AI Can Enhance Enterprise Asset Management Processes
The first three AI applications we’ll discuss regard the collection and management of asset-related data.
1. Utilize Optical Character Recognition (OCC) to Scrape Information
The first way artificial intelligence can help your asset management processes is in collecting information about your assets in the field. To do this, some solutions leverage optical character recognition (OCC), enabling you to simply take a picture of your asset tag, nameplate, or label.
From there, OCC uses machine learning and pattern recognition to scrape key pieces of information from the plates and present the information to you on your device. Depending on the sophistication of the OCC, it can do this with even the rustiest of plates, making it an invaluable tool when plates may not be legible to the human eye.
By using OCC, organizations can reduce the time it takes to gather information about assets. Instead of jotting down the information on a piece of paper (which could take generally around 1-5 minutes), users can snap a photo of a name plate with solution that has OCC capabilities and gather this information in a matter of seconds.
2. Use Machine-Learning To Improve Asset Master Data Consistency
Another way AI can be leveraged in asset management is by using machine learning to ensure data consistency and accuracy.
To do this, machine learning algorithms can reference asset records, BOMs, materials, and other types of records to identify characteristics that should generally be included in a digital record.
With this baseline of knowledge, it can then scan records to ensure they include pertinent details such as manufacturer name, manufacturer number, short description, long description, UNSPSC code, class, characteristic name, and characteristic value.
If data records are missing a piece of information, the machine learning can flag to item for review and suggest missing information by leveraging similar records from external databases.
Instead of sifting through master data by hand, these AI capabilities enable end users to receive alerts on missing asset information quicker and enable them to review and approve suggested missing pieces of information.
3. Use AI To Cross References Records in Multiple Databases and Maintain Governance Rules
In addition to helping you create consistency and completeness of your asset master data, AI can also be used to check for duplicate and redundant information, as well as to uphold business governance rules.
Similar to the AI capabilities mentioned in the sections above, machine learning is leveraged to identify patterns and scan information to spot items that deviate from the standards it has been instructed to uphold.
Without AI capabilities to govern data, most organizations must maintain and uphold their data governance by word of mouth or through continuous data audits. Unfortunately, this process is time-consuming and has a lower level of accuracy.
With streamlined AI capabilities, organizations can access a standardized way to govern their master data according to their business rules and gain a newfound trust in the accuracy of their master data. Our next three AI use cases target the monitoring and diagnosis of asset operation and reliability issues.
4. Create Predictive Models to Track Asset Performance to Identify Anomalies
The next way AI can be leveraged in asset management is to build the foundation for true predictive maintenance. Machine learning and neural network capabilities can be used to create predictive models based on asset operating behavior.
These models form a pattern for normal operating behavior for each asset and continuous operations. By doing this, it makes it easy for AI to detect small deviations in asset operating behavior.
With predictive models, end users can receive alerts when anomalies occur. Without automated predictive models, end users must manually scan asset operating behavior to detect behavior that falls out of normal operation.
Not only do traditional, non-AI powered methods take more time, they can also prevent the end user from catching the issue in time to prevent it.
5. Leverage Historical Actions to Provide Suggested Actions
The above predictive models focus the human interaction to a small subset of process data; allowing users to ignore the other 99%. However, manually processing 1% of the operating data can still be time-consuming. Another powerful capability of AI is the ability to use classification algorithms to identify historical actions taken by end users to inform future actions.
With this information, the AI capabilities can provide end users with suggested actions the user can take like snooze alert, retrain model, or flag for diagnosis based on how a similar alert was addressed in the past.
This can enable organizations to retain insights from past asset experts even if they are no longer with the organization while streamlining the alert screening process. This type of capability helps bridge knowledge gaps for newer asset managers by providing them with a second opinion through suggested actions.
6. Leverage Historical Behaviors To Provide Diagnostic Guidance
One other way AI can be leveraged in asset management is through using generative AI to provide diagnostic assistance. To do this, generative AI pulls information about assets and their history to provide diagnostic suggestions and assistance to users.
Through generative AI capabilities, users can select diagnostic guidance on asset issue causes, diagnostic actions, information on asset history, information on similar assets, and mitigative actions.
Using historical behavior to help with diagnosis enables teams to increase the efficiency of their issue diagnosis. Like leveraging historical information for suggested actions, leveraging historical behavior for diagnostic assistance can also ease transitions as experienced team members retire.
Discover How You Can Leverage AI in Your Asset Management
Digitally transforming your asset management and leveraging AI in your asset management solutions can enable you to take your enterprise asset management to the next level and maximize your ROI.
Are you interested in learning more about how AI can be used in asset management processes like master data management and asset performance management?
Register for our upcoming webinar “How to Achieve REAL Results with AI in Enterprise Asset Management” on June 26th at 10:00am EST, where we will speak with Verdantix Analyst, Joe Lamming, about trends in AI and how organizations can improve their ROI with AI.