- Who We Serve
- Solutions
- The Prometheus Platform
- Asset Performance Management
- Contractor Management
- Construction & Commissioning Management
- Electronic Permitting
- Environmental Health & Safety
- Master Data as a Service
- Maxavera
- Mobile EAM
- Planning and Scheduling
- Process Safety Management
- Reporting and Analytics
- SAP Master Data Governance
- Shutdown, Turnaround, & Outage
- Your ERP
- The Prometheus Platform
- Pricing
- Services
- Resources
- Company
Who We Serve
Solutions
Prometheus Platform
Asset Performance Management
Contractor Management
Capital Project Management
Electronic Permitting
Environmental Health & Safety
Master Data as a Service
Maxavera
Mobile EAM
Planning and Scheduling
Process Safety Management
Reporting and Analytics
SAP Master Data Governance
Shutdown, Turnaround, & Outage
Resources
Company
Life at Prometheus
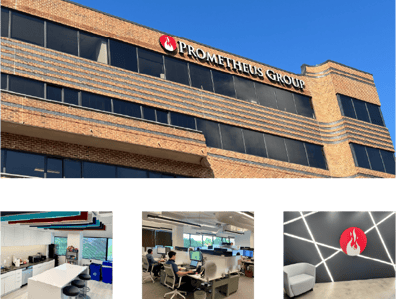